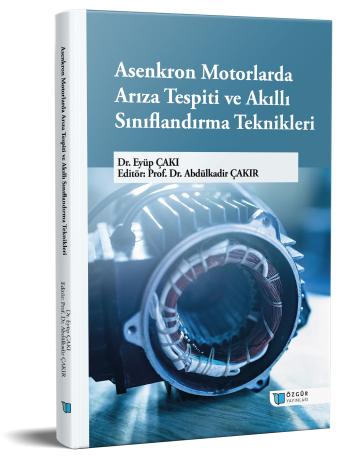
Fault Detection and Intelligent Classification Techniques in Induction Motors
Synopsis
This book comprehensively addresses the fault detection and diagnostic processes in induction motors, widely used in industrial applications. The study focuses on detecting, classifying, and preventing faults such as rotor bar failures, axial misalignments, and bearing issues. The text provides a detailed analysis of machine learning and artificial intelligence-based methods, such as Support Vector Machines (SVM), Artificial Neural Networks (ANN), and ensemble approaches like Random Forest and XGBoost, highlighting their advantages in fault classification and prediction.
The work emphasizes the importance of dimensionality reduction techniques, such as PCA and LDA, in improving computational efficiency and reducing overfitting risks. It also explores the significance of real-time applications, IoT integration, and cloud-based analytics for seamless and efficient fault monitoring in dynamic industrial environments. Predictive maintenance strategies are discussed extensively, integrating time-series analysis and advanced classification algorithms to optimize machine health monitoring and maintenance schedules.
Additionally, the book examines the challenges of working in noisy environments, addressing issues like sensor fusion and ensemble techniques to handle incomplete or noisy data effectively. Performance metrics, including accuracy, precision, recall, F1 score, and AUC-ROC, are thoroughly evaluated to ensure robust algorithm assessment. The comparative study of various algorithms, such as Naive Bayes, SVM, ANN, and Random Forest, reveals their strengths and limitations concerning speed, memory usage, and scalability.
This comprehensive review of fault detection methodologies combines theoretical insights and practical case studies from various industrial domains, including automotive, energy, and petrochemical industries. It provides an essential resource for engineers and researchers aiming to advance fault diagnostic systems in induction motors and similar applications.