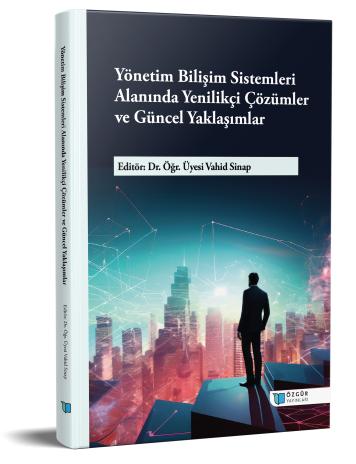
Algorithmic Bias and Ethical Decision-Making from a Management Information Systems Perspective
Chapter from the book:
Sinap,
V.
(ed.)
2025.
Innovative Solutions and Contemporary Approaches in Management Information Systems.
Synopsis
Artificial intelligence (AI) and data-driven decision support systems have transformed decision-making processes at both corporate and societal levels, enabling more efficient and rapid outcomes. However, algorithmic bias has emerged as a critical ethical and governance issue. Imbalances in training data, modeling choices, and the lack of transparency in decision support systems (DSS) can systematically produce unfair outcomes for specific groups. In critical domains such as finance, human resources, healthcare, and law, the integration of algorithms into data-driven decision-making processes aims to enhance objectivity. Yet, biased modeling processes pose a significant risk of reinforcing existing inequalities rather than mitigating them. The purpose of this study is to examine algorithmic bias under the categories of data bias, model bias, and bias in decision support systems, evaluate it through decision-making theories (bounded rationality, prospect theory, and DSS theory), and propose strategic recommendations for establishing ethical, accountable, and transparent decision support mechanisms. From the perspective of Management Information Systems (MIS), algorithmic bias is not merely a technical problem but also a governance challenge directly linked to the ethical responsibilities of organizations. In this context, the Fairness, Accountability, and Transparency (FAT) framework plays a crucial role in ensuring that algorithms become fair, accountable, and transparent, thereby enhancing the reliability of decision-making mechanisms. Developing corporate-level algorithmic audit mechanisms, promoting explainable AI (XAI) techniques, and adopting governance models based on ethical regulations are fundamental solution areas for managing algorithmic bias and fostering fair AI applications. In conclusion, algorithmic systems must not only be optimized technically but also supported by ethical and managerial frameworks to ensure the widespread adoption of responsible AI within the scope of MIS, making it an essential necessity.