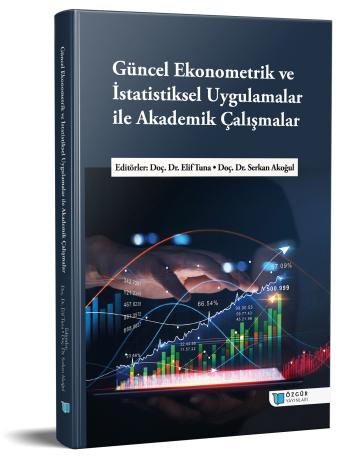
LSTM-Based Multivariate Deep Neural Networks for Stock Price Forecasting
Chapter from the book:
Akoğul,
S.
&
Tuna,
E.
(eds.)
2024.
Academic Studies with Current Econometric and Statistical Applications.
Synopsis
The inherent complexity and dynamic nature of financial markets present substantial challenges for accurately forecasting price movements. Traditional forecasting models often struggle to capture these intricacies, leading to suboptimal predictive performance. In this context, deep learning offers a promising alternative due to its ability to process and analyze large volumes of financial data. This chapter aims to forecast the one-day-ahead price of a Turkish stock over the period from July 30, 2007, to October 11, 2023, using multivariate inputs, including the closing price of the XU030 index, Brent crude oil price, gold price per ounce, the difference between intraday high and low prices, the difference between intraday closing and opening prices, and the ratio of the 14-day to 60-day moving averages. All price-based variables are denominated in U.S. dollars using the TRY/USD exchange rate to ensure consistency.
Using this multivariate dataset, the study employs a deep neural network framework, incorporating Vanilla Long Short-Term Memory (LSTM), Stacked LSTM, Bidirectional LSTM (Bi-LSTM), Convolutional Neural Network-LSTM (CNN-LSTM), and Convolutional LSTM (ConvLSTM) models, with lagged input variables spanning up to five days. A review of the literature highlights that most existing studies focus primarily on univariate forecasting of stock prices or market indices, while fewer address multivariate time series using deep neural networks. The results indicate that, among the models tested, the Vanilla LSTM achieves the highest accuracy in one-day-ahead price forecasting, with a Mean Absolute Percentage Error (MAPE) of 2.20%, demonstrating its robustness in handling multivariate data.