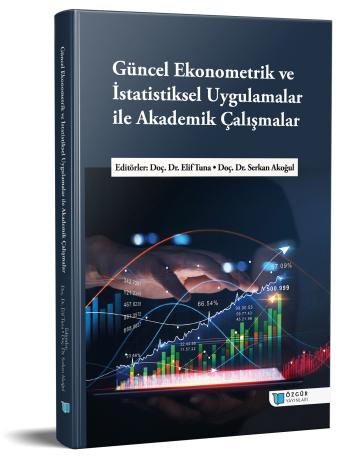
Bi-Rads Classification in Mammography using Deep Learning
Chapter from the book:
Akoğul,
S.
&
Tuna,
E.
(eds.)
2024.
Academic Studies with Current Econometric and Statistical Applications.
Synopsis
This paper presents a deep learning-based procedure that employs the BI-RADS (Breast Imaging Reporting and Data method) classification system on the mammography images. The proposed procedure has the potential to make breast cancer identification and diagnosis more precisely and provide a decision support system for the radiologists. Using RSNA and Vindir mammogram datasets, BI-RADS classification models were estimated by InceptionResNetV2 architectures where they achieved 91% precision and 80% F1-score for BI-RADS 0 classification. Specifically, our proposed comprehensive preprocessing pipeline consists of image cropping, gray thresholding and histogram equalization to enhance the feature visibility and model performance significantly. The findings obtained from analysis results show that deep learning based InceptionResNetV2 architecture achieved 91% precision for BI-RADS classification. Also, our findings contribute to the literature on AI-based medical imaging in terms of addressing important issues in the preprocessing of medical images and enhancing the performance of deep neural networks. As a result, these findings show that deep learning might help the experts for the clinical decision-making in breast cancer diagnosis and provide a decision support system.