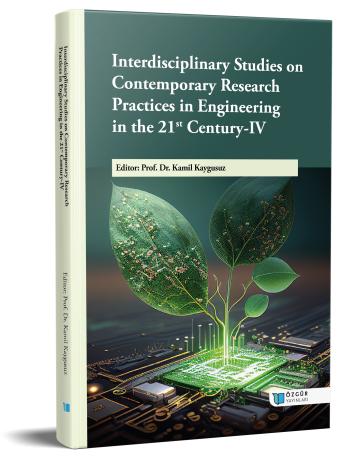
Data-Driven Predictive Modeling of Cylinder Pressure: A Comparative Analysis of Gaussian Process Regression, Artificial Neural Networks, and Ensembles of Trees
Chapter from the book:
Kaygusuz,
K.
(ed.)
2023.
Interdisciplinary studies on contemporary research practices in engineering in the 21st century-IV.
Synopsis
Fossil fuels have traditionally played a crucial role in global energy production, but they cause significant environmental challenges. In response, the scientific community has shifted its focus toward renewable alternative fuels. In this context, biodiesel and alcohols have emerged as promising options for diesel engines. This study is centered on predicting the cylinder pressure of a single-cylinder four-stroke diesel engine fuelled with a diesel fuel-biodiesel (methyl ester)-isopropanol ternary blend using three machine learning algorithms: Gaussian Process Regression (GPR), Artificial Neural Networks (ANN), and Ensembles of Trees (ET). The cylinder pressure data is collected under the full throttle condition and different engine speeds. GPR, ANN, and ET algorithms are trained and compared using root mean square error and regression analysis. GPR exhibits outstanding prediction performance during the validation, with a lower root mean square error of 0.12686, and r2 of 1.00. ANN also exhibits strong prediction performance, with a validation of root mean square error of 0.47081, and a r2 of 1.00. ET, while showing a slightly higher validation root mean square error of 1.73370, maintains strong predictive capability with an r2 of 0.99. However, a comparison between the measured cylinder pressure data and the predicted values reveals a qualitatively and quantitatively closer agreement, particularly for ANN. These findings can suggest the practicality and reliability of these algorithms for predicting cylinder pressure in internal combustion engine studies. In conclusion, this study can contribute to the expanding body of research on alternative fuels and machine learning applications in internal combustion engines.